IAMBEE's Aims
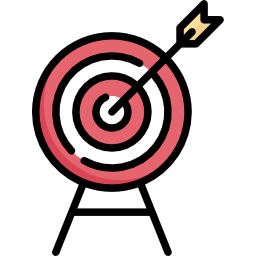
IAMBEE is a tool that aims at identifying adaptive mutations/pathways based on the genomics analysis microbial populations that display the same focal endpoint (adapted phenotype).
Parallel evolution to identify adaptive mutations
Experimental evolution helps understanding how genomic variation contributes to the acquisition of interesting traits (e.g. higher ethanol tolerance). Evolution experiments start from a single clone cultivated for several generations under predefined selective conditions. Fitness is traced over time and the evolved population and/or a selected set of adaptive clones are genotyped. Variants that are fixed in the genomes of the adaptive clones and that were not present in the ancestral strains (or variants that reach high frequency in the evolved populations) can be prioritized as potential causal mutations (here referred to as adaptive mutations).
However, not all high frequency variants fixed in the evolved population are causal: also neutral or slightly deleterious mutations can hitchhike to fixation e.g. when preceding the causal mutations. These hitchhiking mutations are referred to as passengers. The difficulty in the analysis of an experimental evolution experiment is the distinction between adaptive and passenger mutations. A higher mutation frequency in the population results in an increased ratio of passengers to adaptive mutations, further complicating their distinction.
To filter passenger from causal mutations, the experimental set up is extended by including parallel evolving populations. The idea is that because of parallel evolution, the causal mutations will independently be selected for in each of the evolved populations whereas the passenger mutations occur randomly. By counting the frequency with which individual mutations or mutated gene occur in the independently evolved adapted populations, one can try to better distinguish the adaptive from the passenger mutations. The larger the number of independently evolved populations, the higher the power of this frequency-based approach. However, frequency-based approaches are often underpowered in microbial evolution experiments, because of the limited number of parallel evolved populations that are assessed in most experimental evolution experiments, but also because the intrinsic evolutionary properties of clonal systems. In independently evolving clonal populations, the same observed phenotype can occur by hitting a crucial causal pathway. However, as all populations evolve independently there are many different ways in which the same pathway can be hit, through different mutations in different genes. As a result, there is no guarantee that exactly one causal mutation or the same mutated gene will reoccur in the different assessed parallel populations.
IAMBEE takes into account the intrinsic evolutionary properties of clonal systems by searching for consistently mutated molecular pathways rather than consistently mutated genes.
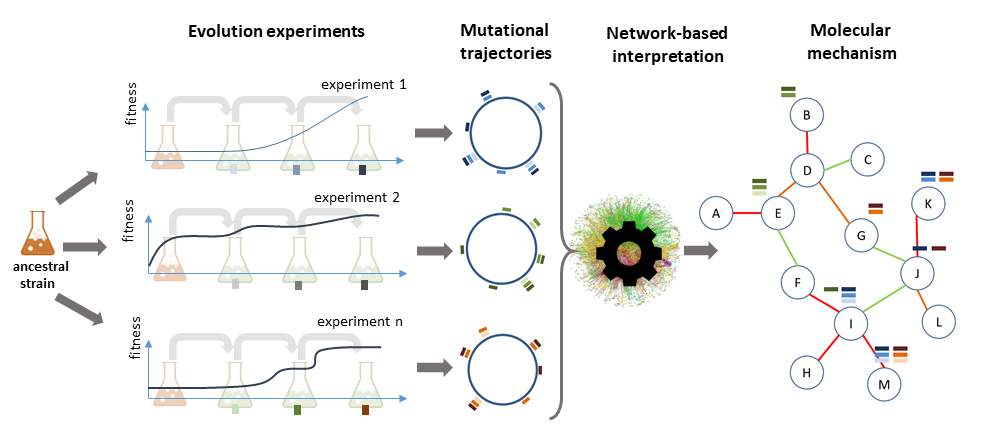
Setup and data analysis flow: An ancestral strain is evolved in parallel under a preset selection pressure. Mutations arise randomly in each of the evolved populations. If a mutation is beneficial, the cells carrying that mutations are fitter and increase in frequency. Clones carrying these mutations start dominating the population (selection sweep). The overall fitness of the population (blue lines) increases accordingly. Note that the mutations in the different parallel evolved lines that result in a selection sweep are not necessarily identical between the different lines. However, chances are high that adaptive mutations hit the same pathways (referred to as driver pathways). The principle of IAMBEE is to identify these recurrently mutated pathways.
IAMBEE: Network-Based Identification of Adaptive Pathways
To search for recurrently mutated pathways, IAMBEE relies on a network-based approach. Rather than using predefined pathway definitions, IAMBEE searches for subnetworks on an interaction network that are recurrently mutated. The analysis is thus driven by an interaction network.
To steer its search for these recurrently mutated sub-networks, IAMBEE exploits additional properties of the evolution trajectories and mutations to weight the impact of the observed mutations and populations during the search for recurrently mutated sub-networks.
IAMBEE assumes that not all mutations are equally likely to be causal to the phenotype. To prioritize the most likely mutations, IAMBEE assumes that true adaptive mutations follows the following:
- Are likely to increase in frequency during a selective sweep.
- Have a larger functional impact
In addition, IAMBEE assumes that populations with higher mutations rate, should contribute relatively less information to the identification of recurrently mutated sub-networks: Populations with high mutation rate accumulate relatively more passengers than drivers. Hence, the chance that a passenger mutation coincidentally hits a true driver pathway increases with the population’s mutation rate. IAMBEE thus explicitly compensates for differences in mutational frequencies between the evolved populations when searching for recurrently mutated sub-networks.
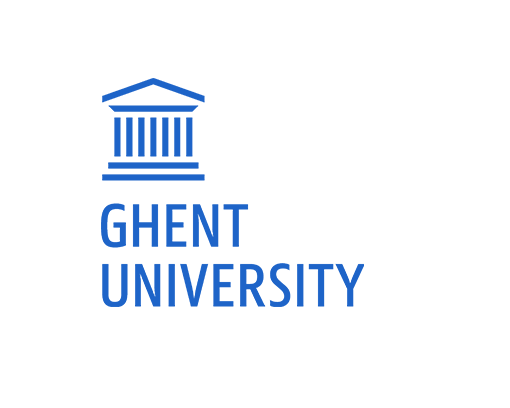